
Driverfinder Archives

driverfinder Archives
Category: Driver
Internet Download Manager

DriverFinder for Windows
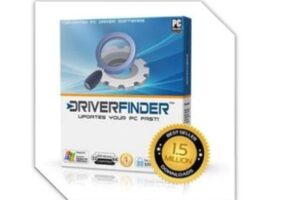
Surface Pro 7 Drivers

Canon canoSCAN LiDE 80 Driver

Canon LiDE 110 Driver Download

DriverFinder: A Gene Length-Based Network Method to Identify Cancer Driver Genes
Abstract
Integration of multi-omics data of cancer can help people to explore cancers comprehensively. However, with a large volume of different omics and functional data being generated, there is a major challenge to distinguish functional driver genes from a sea of inconsequential passenger genes that accrue stochastically but do not contribute to cancer development. In this paper, we present a gene length-based network method, named DriverFinder, to identify driver genes by integrating somatic mutations, copy number variations, gene-gene interaction network, tumor expression, and normal expression data. To illustrate the performance of DriverFinder, it is applied to four cancer types from The Cancer Genome Atlas including breast cancer, head and neck squamous cell carcinoma, thyroid carcinoma, and kidney renal clear cell carcinoma. Compared with some conventional methods, the results demonstrate that the proposed method is effective. Moreover, it can decrease the influence of gene length in identifying driver genes and identify some rare mutated driver genes.
1. Background
At present, understanding the mechanisms of cancer development and uncovering actionable target genes for cancer treatment are still difficult challenges. With rapid advances in high-throughput sequencing technologies, some large-scale cancer genomics projects, such as The Cancer Genome Atlas (TCGA) [1] and International Cancer Genome Consortium (ICGC) [2], have produced different omics data including a rich dataset of whole-exome and RNA sequence data [3, 4], which provides chances to allow us to accurately infer tumor-specific alterations [5] and help in precision medicine in cancers treatment [6, 7]. However, many of genetic changes represent neutral variations that do not contribute to cancer development which are called passenger mutations [6, 8]. Only a few alterations are causally implicated in the process of oncogenesis and provide a selection growth advantage which are referred to as driver mutations [8, 9]. Hence, it is a major challenge to distinguish pathogenic driver mutations from the so-called random mutated passenger mutations [10].
Previously, there were multiple computational methods to identify driver genes based on gene mutational frequency (termed as frequency-based method) in a large cohort of cancer patients [11–13]. However, the infrequently mutated drivers are inclined to be ignored by frequency-based methods. Also mutational heterogeneity in cancer genomes is an important factor affecting the performance of frequency-based methods [14]. In addition, further studies have realized that driver mutations or genes disrupt some cellular signaling or regulatory pathways which promote the progression of cancer [15, 16]. In fact, genes affect various biological processes by related complex networks instead of acting in isolation in cancer [17]. In addition, the cancer is a result of interplay of various types of genetic changes which form complex and dynamic networks [18]. Thus, many network-based and pathway-based approaches have been proposed to prioritize driver mutations and genes. For instance, Dendrix was a pathway-based algorithm for discovery of mutated driver pathways in cancer using somatic mutation data [19]. After that, Multi-Dendrix algorithm was proposed to extend Dendrix method in order to guarantee yielding the optimal set of pathways [20]. MDPFinder was also a pathway-based method to solve the so-called maximum weight submatrix problem proposed in Dendrix method [19] which was aimed at identifying mutated driver pathways from mutation data in cancer [21]. And Zhang et al. proposed CoMDP method which focused on cooccurring driver pathways rather than single pathway [22]. In addition, iMCMC was a network-based method by integrating somatic mutation, CNVs, and gene expressions without any prior information [6]. Another method, DawnRank, was also a network-based algorithm to discover personalized causal driver mutations by ranking mutated genes according to their potential to be drivers based on PageRank algorithm [23]. Bashashati et al. developed a method called DriverNet which comprehensively analyzed genomes and transcriptomes datasets to identify likely driver genes in population-level by virtue of their effect on mRNA expression networks and also reveal the infrequent but important genes and patterns of pathway [10]. VarWalker was a personalized network-assisted approach to prioritize well-known, infrequently mutated genes and interpret mutation data in NGS studies [24].
Although some proposed methods can determine potential drivers, most of them do not consider the influence of gene length to the results; in other words, they may identify some likely false positive driver genes according to known driver genes datasets. And it has been indicated that driver genes are related to not only mutation frequency, but also mutation context or gene length [25] and variants tend to arise more frequently in long genes [26]. For example, TTN, the longest gene in human genome, accumulates many variants just due to its length [24, 26]. TTN may be selected in many computational methods; however, it usually serves as passenger gene [27]. This phenomenon indicates that many current methods have a strong preference towards identifying long genes [24]. So it is essential to filter those frequently mutated genes due to long length. VarWalker takes into account the gene length; however, it does not consider the influence of mutation to expression. In addition, some genomic variations in a gene may lead to extreme changes in some outlying genes expression level which are associated with the mutated gene through gene-gene interaction network or pathways and these outlying genes are often called outliers [10]. And, it has been proved that cancer-associated genes are more effectively detected by interindividual variation analysis rather than only calculating differences in the mean expression across different samples [28]. That is, the outliers are related to not only tumor expression distribution but also the corresponding normal expression distribution. Moreover, various cellular processes are often affected by genes in complex networks rather than genes acting in isolation [17] and cancer is also related to a set of genes interacting together in a molecular network [29]. So networks usually provide a convenient way to explore the context within which single gene operates [30]. It should be noted that prior knowledge such as protein-protein interaction (PPI) network can provide some useful information; however, prior knowledge is limited and may lead to discarding some important information in some instances [22]. In our previous work [31], we only consider the prior information of gene-gene interaction network. So it is essential to extend gene-gene interaction network.
In this study, we proposed an integrated framework named DriverFinder to identify driver genes by integrating somatic mutation data, copy number variations (CNVs), tumor and normal expression data, and gene-gene interaction network. Firstly, the gene length is taken into consideration to filter some frequently genes because of long length. Moreover, in this method, we integrated tumor expression and normal expression to construct outlier matrix rather than only using tumor expression. Furthermore, to increase accuracy of identifying drivers, we calculated Pearson correlation coefficients (PCC) of genes and combined them with PPI network to construct a new dynamic interaction network for each cancer type. In order to estimate the performance of DriverFinder method, we applied it to four different large-scale TCGA datasets, including breast cancer (BRCA), head and neck squamous cell carcinoma (HNSC), thyroid carcinoma (THCA), and kidney renal clear cell carcinoma (KIRC), and compared it with MUFFINN [32], DriverNet [10], and frequency-based method. The results demonstrated that DriverFinder can identify drivers effectively and decrease false positive, that is, filtering some long and frequently mutated but functionally neutral genes.
2. Materials and Methods
We proposed DriverFinder to identify cancer driver genes by integrating multiomics data (Figure 1). The detailed description of it is shown in Figure 1.
As is shown in Figure 1, the first step is to estimate the occurrence of mutation events in the genome by fitting them into a generalized additive model [24]. Then a weighted resample-based test is used to filter long passenger genes according to approximated probabilities based on benchmark of coding gene length. Secondly, for gene expression, we compare expression of tumors with normal samples to determine the outlying genes. Then a gene-gene interaction network combined with prior knowledge and PCC among expression data is used to relate mutations to their consequent effect on gene expression. The associations between mutated and outlying genes are formulated using a bipartite graph where the left nodes indicate the genes mutation status and the right nodes indicate the outlying expression status in each patient. For each patient, there is an edge between and if the left partition gene is mutated and the right gene is an outlier in some samples and they also have high correlations in gene-gene interaction network. Secondly, greedy algorithm is used to prioritize mutated genes based on the coverage. In each iteration of the greedy algorithm, the mutated gene on the left partition of the bipartite graph which relates to the most outlying expression genes is chosen. Until all the outlying expression genes are covered by the least mutated genes on the left, iteration is stopped. Then the mutated genes are ranked based on their coverage. So genes with the most outlying expression are appointed as candidate driver genes. Finally, the statistical significance test based on null distribution is applied to these putative driver genes.
2.1. Construction of Mutation Matrix
In terms of somatic mutation, we downloaded it from TCGA data portal (https://cancergenome.nih.gov/) and only considered the data of level 2. As a matter of fact, Jia et al. explored long genes in two datasets and examined gene length effects by plotting the proportion of mutated genes versus their complementary DNA (cDNA) length [24]. They discovered that two sets of mutated genes were positively correlated with cDNA length, and longer genes were more likely to be mutated genes [24]. Hence, frequency-based methods may be inclined to select long genes as drivers. So, it is necessary to perform gene length-based filtration. In this study, in order to accurately estimate the mutation rate for each gene, generalized additive model was adopted to compute a probability weight vector (PWV) for the mutation genes of each sample [24].
Here, only somatic mutated genes mapped onto the benchmark of consensus coding sequences (CCDS) dataset [33] which contains a core set of consistently annotated and high quality human and mouse protein coding regions are reserved. And those mapped genes in this study have been allocated cDNA length based on their coding sequences [24]. Assuming the vector as the cDNA gene length and the following model is used to assess the probability of a gene to be mutated, where represents an unspecified smooth function and represents the proportion of mutant genes in the specific samples [24]. Each gene then would be assigned a PWV value. Afterwards, a resampling test based on the probability of each gene is performed 1000 times in each sample and the null distribution is that in genes mutations occur at random. Then we define mutation frequency aswhere represents mutation frequency. Next we filter out genes with frequencies ≥ 5% in random datasets unless they are Cancer Gene Census (CGC) genes. Then a list of significant mutant genes is obtained.
As for CNVs, which have been processed by GISTIC 2.0, they are acquired from http://gdac.broadinstitute.org/runs/ (v2014_10_17). There are five types of copy number including amplification, gain, diploid, heterozygous deletion, and homozygous deletion in this dataset. Here, we only screen out amplifications and homozygous deletions to construct CNV matrix . Finally, the significant mutated gene list and CNV matrix are combined to generate the patient-mutation binary matrix , in which indicates there is genetic alteration, that is, mutation, amplification, or homozygous deletion, in the jth gene of the th sample. Otherwise, .
2.2. Construction of Expression Outlier Matrix
Gene expression data (level 3) including tumor and normal expression data is also downloaded from TCGA data portal. Moreover, some studies have shown that assessment of interindividual variation of gene expression performs well in predicting cancer-associated genes [28]. So in this study, the outlying matrix is determined based on analysis of interindividual variation in tumor and normal expression rather than differences in mean expression levels or only tumor expression distribution [28]. For each type of cancers, there are two expression datasets and which indicate the real-valued expression measure of gene in sample of tumor and normal datasets, respectively. For each gene, the outliers in this study are defined as tumors whose expression levels are outside the four-standard deviation range of the expression values of the gene across all the normal samples [28]. It is formularized asin which is the mean expression and indicates the standard deviation of gene expression in normal samples. Then the binary patient-outlier matrix is constructed and the value of indicated whether gene in patient is an outlier among the population-level distribution for that gene. If the expression of gene is an outlier in patient , ; otherwise, .
2.3. Gene-Gene Interaction Network
It is noteworthy that most prior knowledge such as PPI network or pathways is incomplete and a great deal of knowledge about biological pathways remains unclear [22]. In our previous study [31], we relied on prior knowledge about gene influence graph integrated from known gene networks [10] which often leaved out some likely important nodes. So in this study, we constructed a new dynamic gene-gene interaction network by incorporating gene-gene correlation coefficients with prior knowledge. That is, firstly, PCCs between pairwise genes are obtained by normalized tumor expression. Acceptable correlations with PCC > 0.75 are often considered high correlated and selected [34]. Here, we choose 0.8 as threshold to ensure selected pairwise genes being higher correlated and increase reliability. The edges with PCC > 0.8 are selected and set to 1, otherwise 0. In order to retrieve some important prior knowledge simultaneously, the known gene network (termed the influence graph in DriverNet [10]) is mapped onto the binary matrix obtained from correlation coefficients matrix. So a new and dynamic gene-gene interaction network (termed as after) included prior knowledge and deduced knowledge is established. When there is a correlation, that is, PCC > 0.8 or 1 in influence graph between gene and gene , ; otherwise, .
2.4. Significance Estimation
With the aim of testing the statistical significance of the driver candidates, we apply a randomization framework. The algorithm is run on the random permuted original datasets (mutation data and outlier data). Then we assess the significance by seeing if the results on real data are significantly different from the results on random datasets and obtain the value of each candidate drivers. The statistical significance of is defined as follows [10]:
DeskToolsSoft
Driver update utility DriverFinder certified as compliant, clean software by AppEsteem
San Francisco, CA – September 5, 2019 – DeskToolsSoft, a leader in computer optimization tools and solutions development, has just announced the AppEsteem certification of DriverFinder, a driver scanning, downloading and updating utility for Windows-based PCs.
“Our motto at DeskToolsSoft is ‘PC Software Solutions Done Right’ and that’s exactly what we’ve proven by having DriverFinder reviewed, tested, and certified by AppEsteem. This confirms our commitment that consumers come first, and that we do that by creating only responsible, clean software for them to use”, according to DeskToolsSoft representative Melanie Tan.
“We are also extremely proud of the fact that DriverFinder passed AppEsteem’s rigorous review and approval process… at first pass.”
Founded and led by former cybersecurity executives at Microsoft®, AppEsteem rigorously reviews, tests and certifies software and related services to ensure that consumers can download and install programs without fear. AppEsteem guidelines, created in close consultation with security experts, software platforms, software vendors, government regulators and consumer groups, are considered to be the most comprehensive consumer-protection guidelines in the software download industry today.
With the updated and certified version of DriverFinder, users are assured that the software they are downloading is clean, free of malware, free of any virus, and is completely transparent. It ensures that anyone using DriverFinder will NOT be subjected to any unethical software practices such as deceptive bundling, undisclosed installations, and exaggerated claims.
The certifcation reinforces that DriverFinder is clean to use, safe to use, and fulfills its purpose – find and update Windows® drivers for PC users – simply and accurately.
DriverFinder 3.8.0 is available for immediate download at its official website: https://www.driverfinderpro.com
Microsoft, Windows, Internet Explorer and Microsoft Edge are either registered trademarks or trademarks of Microsoft Corporation in the United States and/or other countries. No affiliation or endorsement is intended or implied.
AppEsteem Certification for DriverFinder:
https://customer.appesteem.com/certified?vendor=DESKT
About DeskToolsSoft, Inc.
DeskToolsSoft, backed by more than 20 years of experience in the IT industry, was established with one aim: to provide desktop utilities that do exactly what ‘it says on the box’; namely, to optimize computers on various, specific levels so that users get the most performance out of their machines.
The DeskToolsSoft goal is not only to produce software to help clients with system maintenance but to produce software that UPLIFTS their computing experience. DeskToolsSoft achieves this by utilizing only the best processes and technology in the IT industry, and presenting them in a highly user-friendly interface for clients.
Media Contact:
Diana McCord
DeskToolsSoft, Inc.
Email: media (at) desktoolssoft (dot) com
Phone: (408) 600-1418
http://www.desktoolssoft.com
###
What’s New in the driverfinder Archives?
Screen Shot

System Requirements for Driverfinder Archives
- First, download the Driverfinder Archives
-
You can download its setup from given links: